📊 Performance
- The risk premium in Treasury bonds varies more frequently than business cycles.
- A new factor ("cycle factor") explains excess bond returns better than traditional forward rate models.
- The model outperforms Cochrane-Piazzesi’s single-factor return predictor in and out of sample.
đź’ˇ Key Idea
The paper introduces a "cycle factor" to decompose Treasury yields into inflation expectations and a risk-premium component. This approach enhances return forecasting and outperforms traditional models that rely solely on forward rates.
📚 Economic Rationale
Most term-structure models focus on level, slope, and curvature. The authors instead emphasize economic drivers:
- Expected Inflation (trend inflation).
- Real Short Rate (business-cycle component).
- Risk Premium (new "cycle factor").
By isolating these components, they show that bond risk premia move at a higher frequency than previously thought.
🚀 Practical Applications
- Improved Bond Return Forecasting – A better predictor of excess bond returns across maturities.
- More Accurate Risk Premium Estimation – Reduces sensitivity to measurement errors in yield data.
- Better Asset Allocation – Helps investors time exposure to Treasury bond risk premia more effectively.
🛠️ How to Do It
Data
- US Treasury yield curve data (1971–2011).
- Inflation expectations from survey and historical CPI data.
- Forward rates & excess bond returns.
Model/Methodology
- Decomposes yields into trend inflation, real short rate, and risk premium.
- Constructs a "cycle factor" to predict excess returns.
- Controls for inflation expectations to separate risk premia from interest rate movements.
Strategy
- Use the cycle factor to time bond allocations – Capture periods of high risk premia.
- Avoid relying only on forward rates – They contain excess noise and do not fully explain returns.
- Monitor real-rate cycles – These provide key signals for bond risk premia shifts.
📊 Table or Figure
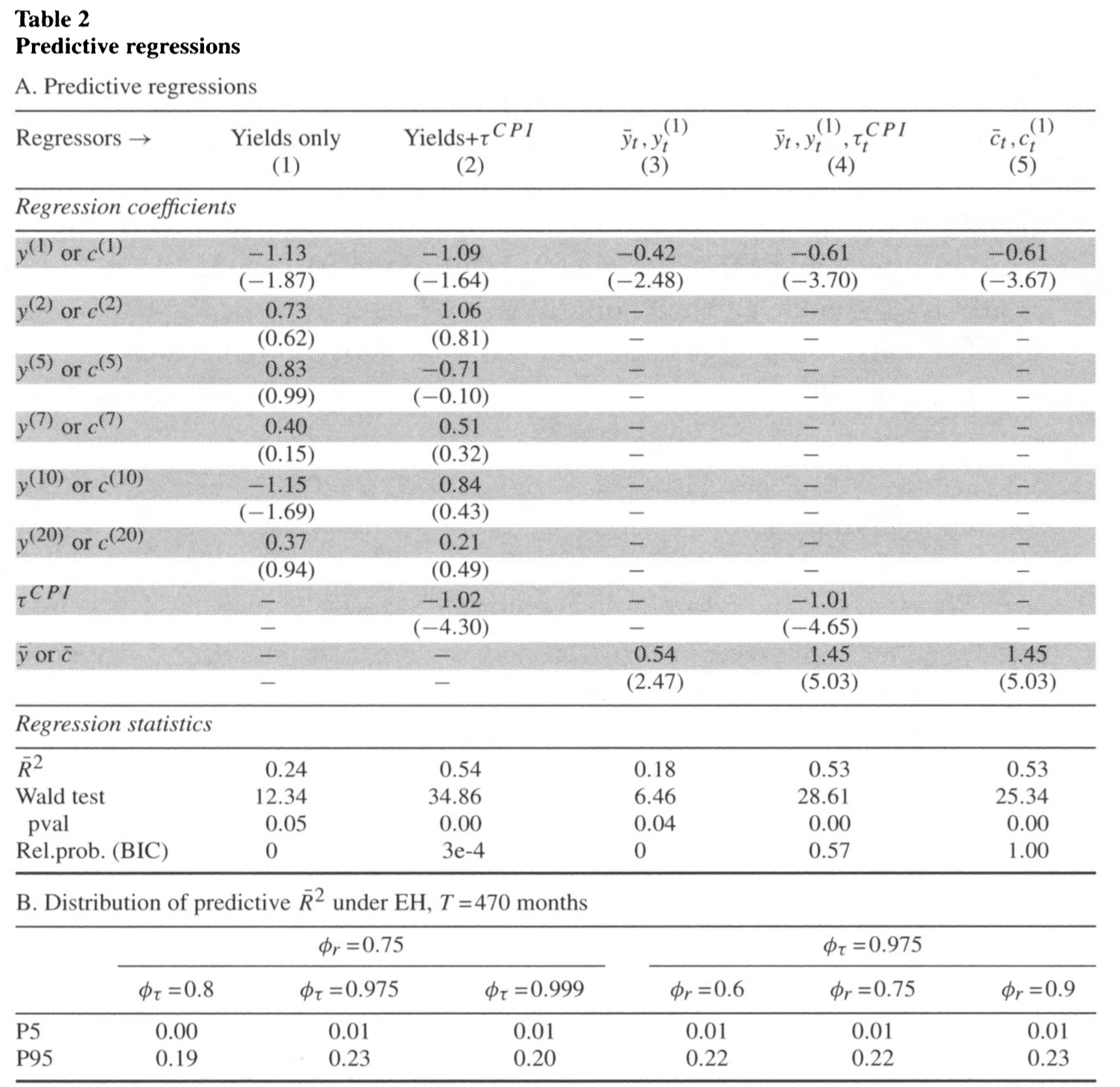
📌 The cycle factor outperforms forward rate models in predicting excess Treasury bond returns out of sample, improving return forecasts by 10-30%.
đź“„ Paper Details
- Authors: Anna Cieslak & Pavol Povala
- Journal: The Review of Financial Studies (2015)
- DOI: 10.1093/rfs/hhv032