Key Performance Metrics
How Well Does This Strategy/Model Perform?
- Sharpe Ratio:
- Linear Attention: 3.89
- Transformer: 4.57
- Pricing Error (HJD):
- Linear Attention: 0.14
- Transformer: 0.09
Takeaway:
📈 The transformer-based AIPM significantly outperforms existing ML models by enhancing cross-asset information sharing and leveraging deeper architectures.
Key Idea: What Is This Paper About?
This paper introduces Artificial Intelligence Pricing Models (AIPMs) that embed transformer architectures into the stochastic discount factor (SDF). It demonstrates that sharing information across assets and scaling model complexity improves predictive accuracy and reduces pricing errors in asset returns.
Economic Rationale: Why Should This Work?
Transformers mimic the success of large language models by contextualizing information—here, across stocks instead of words. By embedding asset-level characteristics into a shared space, the model captures richer, nonlinear interactions and conditional dependencies.
Relevant Economic Theories and Justifications:
- Contextual Learning: Just like words in context, stocks benefit from cross-sectional information to improve signal quality.
- Factor Timing: The model times characteristic-managed portfolios dynamically, enhancing alpha.
- Virtue of Complexity: Consistent with theory and empirical results, more complex models perform better under large data regimes.
Why It Matters:
Capturing nonlinear, high-dimensional relations across assets breaks traditional modeling limits and unlocks hidden predictive signals.
How to Do It: Data, Model, and Strategy Implementation
Data Used
- Data Sources: Jensen-Kelly-Pedersen (2023) factor database (132 stock characteristics)
- Time Period: 1963–2022
- Asset Universe: US stocks (NYSE/AMEX/NASDAQ)
Model / Methodology
- Model Types:
- Linear Portfolio Transformer
- Deep Nonlinear Transformer (multi-head attention, stacked transformer blocks)
- Training: 60-month rolling windows, ridge penalty (for linear); Adam optimizer (for deep transformer)
Trading Strategy
- Signal Generation: Transformer outputs conditional weights
w_t = T^{(K)}(X_t)λ
for the SDF. - Portfolio Construction: Risk-efficient SDF portfolio optimized via mean-variance principles
- Rebalancing Frequency: Monthly
Key Table or Figure from the Paper
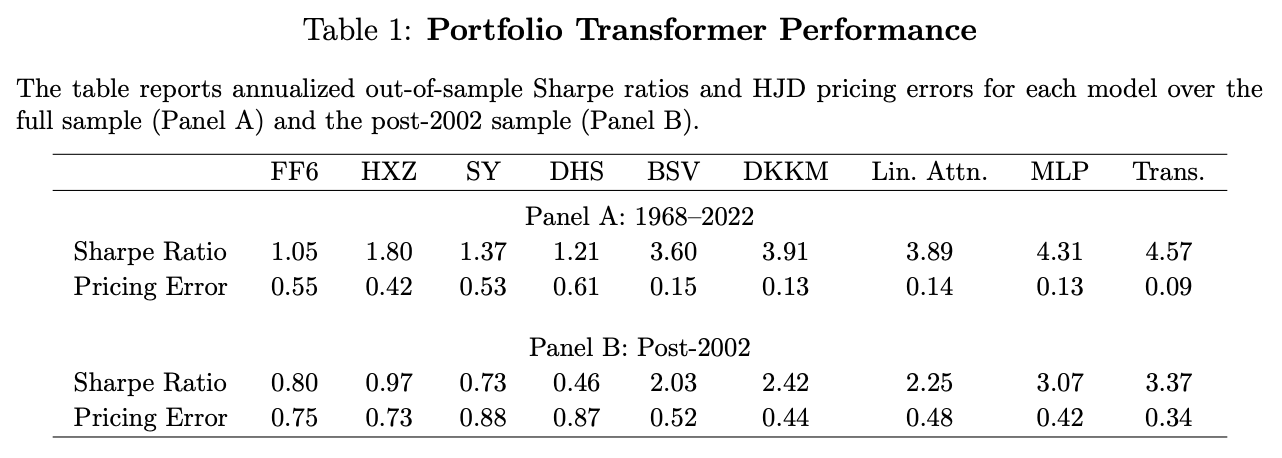
Explanation:
Shows that the nonlinear transformer model achieves a Sharpe ratio of 4.57 and a pricing error of 0.09, outperforming shallow neural networks (e.g., DKKM with SR 3.91, error 0.13) and traditional linear models (e.g., BSV with SR 3.60, error 0.15).
Final Thought
🚀 Transformer-based AIPMs redefine what's possible in asset pricing—context, scale, and complexity deliver real predictive edge.
Paper Details (For Further Reading)
- Title: Artificial Intelligence Asset Pricing Models
- Authors: Bryan T. Kelly, Boris Kuznetsov, Semyon Malamud, Teng Andrea Xu
- Publication Year: 2025
- Journal/Source: NBER Working Paper No. 33351
- Link: http://www.nber.org/papers/w33351